The Scope of Artificial Intelligence in Clinical Oncology
Healthful Vitality | 10/16/2019 | By Dr. Faiq Shaikh, MD | The Scope of Artificial Intelligence in Clinical Oncology
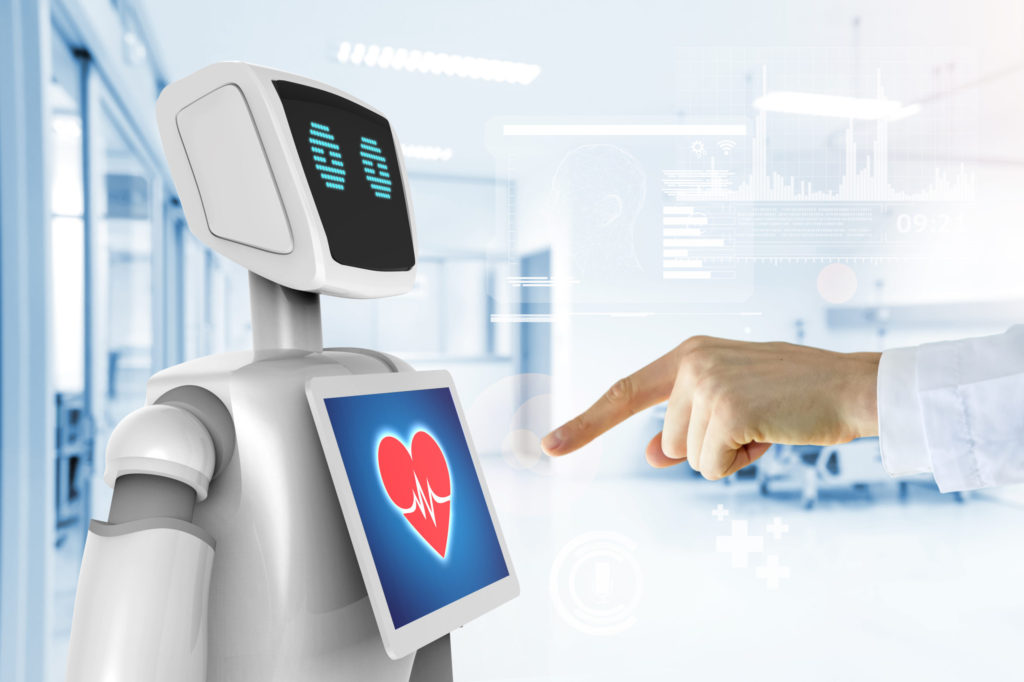
The world of medicine is abuzz with Artificial intelligence (AI) and how it is poised to impact everything from clinical practice to the healthcare business. The field of clinical medicine that has seen the most direct applications of this technology is Oncology. But, in many ways, it has led other specialties of medicine to adopt AI as a technology that transforms clinical management. In this article, we propose to examine the scope of artificial intelligence in clinical oncology.
It is essential to realize that cancers are complex pathological entities. Several genetic and environmental factors contribute to their etiology. As a result, their classification can be complicated. Also, their management is complex, with very high risks involved. These issues make clinical decision-making from diagnosis to treatment and follow-up extremely challenging, requiring a myriad of factors to be considered. Notably, recent advances in computational analysis of radiopathological data has rendered large data sets in oncology. We can process these data sets through Machine Learning (ML) algorithms to help us better understand the natural history of the disease and improve our ability to manage it.
The Scope of Artificial Intelligence in Clinical Oncology: An Overview
Here’s an overview into the various aspects of clinical oncology where AI/ML is making strides:
Disease detection:
In a variety of settings, AI has enhanced our ability to accurately detect cancers and do so in a timely manner. This is especially true in the case of digital pathology, where AI/ML allows us to automate histopathologic pattern detection. Furthermore, molecular pathology techniques (such as FISH, genomic assays, genetic mutation detection) have all benefited from ML approaches. Notably, advanced diagnostic methodologies and AI/ML together have significantly increased our ability to detect cancers earlier and in a more granular way than ever before.
Disease classification:
There is availability of large data sets from different data silos that can be used to train ML models for earlier and more accurate detection of diseases, up to the level of their genetic or histologic subtype, allowing for revised/renewed classifications of several cancers, such as breast cancer. This new approach of classifying disease based on a deeper, truer understanding of cancer subtypes allows us to design better management decision trees for them.
Risk stratification:
A major component of classifying disease is to stratify them in terms of the risk they pose to the patient, in terms of becoming rapidly progressive, anaplastic, locally aggressive, highly metastatic or resistant to therapy. There are huge implications of stratifying cancers this way, as it guides decision making in the key pivot points of disease management. Indeed, ML-driven risk stratification models are a step toward the practice of personalized medicine.
Disease prognostication:
Closely tied to risk stratification is the concept of overall disease prognostication. One of the most appealing uses of AI/ML in clinical medicine is the development of predictive models for disease outcomes. AI/ML enables us to use the overwhelming volume of data available on the disease phenotype and genotype and create predictive models that inform on clinical outcomes.
Malignant transformation assessment:
Another aspect of oncologic disease prognostication is being able to detect or even predict transformation of benign, premalignant conditions to cancer. There are a number of such conditions that have the potential of malignant transformation, including lymphoproliferative disorders (to lymphoma), neurofibromatosis (to neurofibrosarcoma). It is critical to detect this transformation early in its tracks, which typically means that there is a greater likelihood that the available therapeutic options will still be effective. There are many factors that contribute to the overall assessment of these changes, and it can be hard to take them all into account. Hence, AI/ML-given models can consume such variegated data input to provide a score that predicts the likelihood of malignant transformation.
Therapy response prediction:
This is related to the AI’s overall role in predictive modeling in clinical oncology. Cancer treatment is very expensive. It is also very toxic. The clinician has to closely weigh the risks and benefits of each type of cancer treatment available and make the often tough decision on what treatment works best in what period of time in any given patient. The stakes are high – you cannot afford to offer an extremely expensive immunotherapeutic agent, put them on a highly coveted gene therapy trial, or subject them to highly toxic chemotherapy unless you are convinced that the given therapy will truly benefit these patients.
There are hundreds of factors that go into that determination, including the disease genotype, tumor microstructure, metabolome, clinical manifestation, metastatic potential, dedifferentiation, vasculogenesis, just to name a few. On one hand, advanced analytics and more intricate detection methods have made it possible to have such information available at hand, and on the other hand, AI/ML can harness the true power of that information by way of algorithms that can predict the degree of chemo-, immuno- or radiosensitivity of these cancers. This improves the confidence of the clinical oncologist to deliver these therapies to these patients and expect results closer to ground reality.
Therapy response assessment/monitoring:
What may be even more or at least as important as predicting therapy response, is the ability to assess therapy response. As mentioned earlier, these therapeutic options are extremely expensive and with high patient morbidity. Moreover, not all patients respond to them the same way. Therefore, it is very important to separate responders from nonresponders, and do so early on. There is a high cost and morbidity involved in continuing these treatments in patients who are not responding, and conversely, there is justification to continue the treatment in patients who are actually responding. Truly assessing the ground truth is harder than it seems.
The conventional methods of radiologic assessment (RECIST, irRECIST) seem to fall short of doing so, especially confounded by the phenomenon of pseudoprogression. More recent computational analytic approaches, such as Radiomics help us study the deeper tissue-level changes that may be the harbinger of real response status. Indeed, ML allows us to truly harness the power of Radiomics and other -omics to provide us valuable insights to real progression vs regression. This is a hot-button issue in clinical oncology and several academic labs as well as biotech companies are busy developing models that can help us in that determination.
Patient follow-up & surveillance:
Surveilling patients to look for recurrence after remission has been achieved, or in patients in which disease progression has to be tracked, is a significant contributor in the effort and money spent in the clinical oncologic management. This typically involves imaging, serum levels and clinical follow ups. There is a pressing need to develop ML models that can identify the best, most personalized surveillance strategies that are not only cost efficient but also minimize radiation exposure, patient discomfort and associated morbidities.
Conclusion
In conclusion, AI and ML has impacted almost all facets of clinical management of cancer, and that has led to improvements in overall patient care. As we move on to the value-based model of medical practice, using AI-driven approaches will help us to provide the best possible, personalized medical care in the most cost-efficient manner, leading to overall improved patient outcomes. Indeed, AI applications are yet to investigate in full swing. However, AI research has advanced as a groundbreaking healthcare system strategy.
Read more about AI applications: The Application of Artificial Intelligence (AI) in Medicine and Immunotherapy Weds Artificial Intelligence: All Cancer Patients are Invited.
Further reading:
- Artificial Intelligence, Big Data, and Cancer. Hagop Kantarjian, Peter Paul Yu. JAMA Oncol. 2015 Aug; 1(5): 573–574. doi: 10.1001/jamaoncol.2015.1203. Retrieved from https://pubmed.ncbi.nlm.nih.gov/26181906/
- The diagnostic outcomes of esophageal cancer by artificial intelligence using convolutional neural networks. Yoshimasa Horie, Toshiyuki Yoshio, Kazuharu Aoyama, Syouichi Yoshimizu, Yusuke Horiuchi, Akiyoshi Ishiyama, Toshiaki Hirasawa, Tomohiro Tuchida, Tsuyoshi Ozawa, Soichiro Ishihara, et al. Gastrointest Endosc. 2018 Aug 15 Published online 2018 Aug 15. doi: 10.1016/j.gie.2018.07.037. Retrieved from https://pubmed.ncbi.nlm.nih.gov/30120958/
- Application of Artificial Intelligence-based Technology in Cancer Management: A Commentary on the Deployment of Artificial Neural Networks. Sherbet GV, Woo WL, Dlay S. ANTICANCER RESEARCH 38: 6607-6613 (2018) doi:10.21873/anticanres.13027. Retrieved from https://ar.iiarjournals.org/content/38/12/6607
- Artificial Intelligence Systems as Prognostic and Predictive Tools in Ovarian Cancer. A. Enshaei, C. N. Robson, R. J. Edmondson. Ann Surg Oncol. 2015 Nov; 22(12): 3970–3975. Published online 2015 Mar 10. doi: 10.1245/s10434-015-4475-6. Retrieved from https://pubmed.ncbi.nlm.nih.gov/25752894/
- Predicting lung cancer prognosis using machine learning. Burki TK. Lancet Oncol. 2016 Oct; 17(10): e421. Published online 2016 Aug 25. doi: 10.1016/S1470-2045(16)30436-3. Retrieved from https://pubmed.ncbi.nlm.nih.gov/27569440/
- Use of Artificial Intelligence and Machine Learning Algorithms with Gene Expression Profiling to Predict Recurrent Nonmuscle Invasive Urothelial Carcinoma of the Bladder. Georg Bartsch, Jr, Anirban P. Mitra, Sheetal A. Mitra, Arpit A. Almal, Kenneth E. Steven, Donald G. Skinner, David W. Fry, Peter F. Lenehan, William P. Worzel, Richard J. Cote. J Urol. 2016 Feb; 195(2): 493–498. Published online 2015 Oct 13. doi: 10.1016/j.juro.2015.09.090. Retrieved from https://pubmed.ncbi.nlm.nih.gov/26459038/