The Application of Artificial Intelligence (AI) in Medicine
Healthful Vitality | 05/19/2021 | By Dr. Ahinsa Madumali | The Application of Artificial Intelligence (AI) in Medicine.
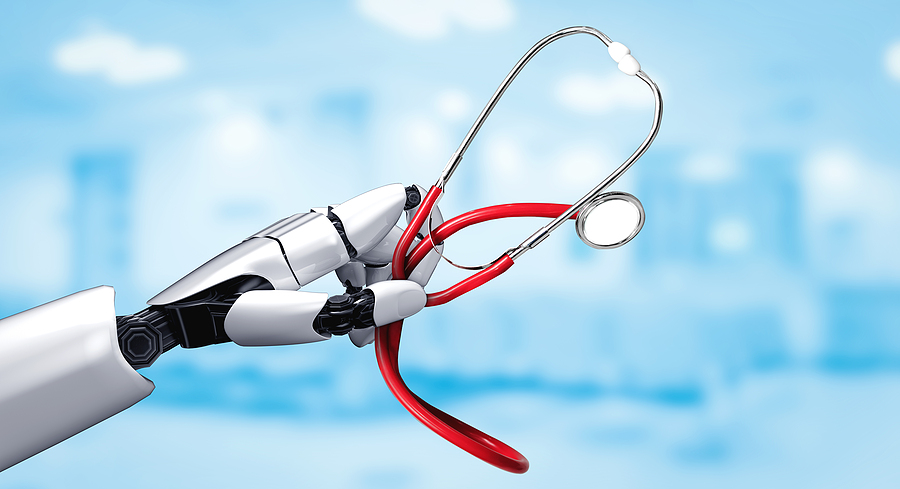
Increasing workloads, the repetitiveness of activities, and struggling with large data sets have stimulated human creativity to innovate numerous machines, systems, and technologies to replace, enhance and exceed human mechanical and logical capabilities. This breakthrough innovation reflects in the application of artificial intelligence (AI) in medicine too. Artificial intelligence (AI) is one of these astonishing inventions, defined as a branch of applied computer science wherein computer algorithms are trained to perform tasks typically associated with human intelligence [1].
AI has started as the simplest “if and then” rules and progressed to use more complex algorithms and larger data sets that are always available in medicine [2]. Utilizing this availability of primary fuels for AI, the medical field has embraced AI in its infancy of evolution to improve the accuracy, efficacy, durability, and strength of medical diagnosis, investigation, treatment, and therapeutic monitoring. Currently, most of the subfields in medicine have engaged in AI as a reliable aid to improve overall patient outcomes and accelerate future directions of the field. Emerging studies show that AI has extensive scope in the use of health and medicine. For example, AI uses in Clinical Oncology, and Immunotherapy, among others.
Evolution of AI in Medicine
It is important to realize the evolution of AI in medicine before we further explore the application of artificial intelligence (AI) in medicine. Alan Turing, in 1950, first described the concept of generating intelligent behavior and critical thinking with the use of computers. Later, the term artificial intelligence was explained by John McCarthy as “the science and engineering of the manufacture of intelligent machines.” In 1976, the first sample of AI application in medicine was developed as a consultation program for glaucoma using the CASNET model and demonstrated the feasibility of AI in medicine.
In 1986, the University of Massachusetts innovated a decision support system called DXplain, which used symptoms to generate a differential diagnosis after processing. Subsequently, AI in medicine began to evolve dramatically. In 2007, IBM created Watson, an open domain question and answer system that won first place in 2011 on the Jeopardy television game show after competing with human participants. Surprisingly, in 2017, IBM Watson successfully identified new RNA-binding proteins that were altered in amyotrophic lateral sclerosis. Currently, AI appears in the medical field continuously with different technologies [2].
Subfields of AI in Medicine
Even this complex technology encompasses many forms of computer science; medicine has mainly focused on the following subfields.
1. Machine Learning (ML)
Programming based on statistical techniques that allows a computer system to recognize patterns and learn from experiences and then to perform without directions and instructions [3, 12]
2. Natural Language Processing (NLP)
Use a computer to understand spoken or documented languages to perform language translation, speech recognition, word processing to extract reliable details. It is widely used to analyze electronic medical records (EMR), medical notes, pharmaceuticals, and medical images [12].
3. Artificial Neural Networks (ANN)
It is a network made up of individual units programmed to perform computer tasks and recognize complex patterns by acting like artificial neurons [12]. This process mimics the human brain, which processes various data types, recognizes and creates patterns through neural networks to make decisions [3].
4. Deep Learning (DL)
A subset of machine learning programmed similarly to human brain processing, which uses multiple data sets simultaneously. During processing, the data is repeatedly evaluated for a different second, third evaluation, and so on until the most appropriate final result is reached [3].
5. Computer Vision
This technology is used for visual search to recognize details and patterns in medical images and videos to identify tumors or malignancy and other abnormalities [12].
6. Convolutional Neural Network (CNN)
A specific type of ANN-based deep learning algorithm used to analyze data that has multiple hidden layers with complex relationships between them [3]
Current Application of Artificial Intelligence (AI) in Medicine
The shortage of clinicians comparatively to the high demand for medical services has created a need for digital solutions to optimize medical resources effectively in cost-effective ways.
1. AI for Medical Data Processing
Automated medical triage systems have been able to help healthcare providers deal with a more significant number of patients in a short period [5]. Vital signs and medical record data utilizing ML systems have been developed as a scoring system for predicting cardiac arrest within 72 h and predicting defibrillation success retrospectively for cardiac arrest after leaving the hospital. Compared with the Emergency Severity Index, ML-based E- triage systems have demonstrated equivalent or improved identification of patient outcomes [6].
2. AI for Medical Image Recognition
AI-based cardiac imaging using CNN and other methods has become popular as they were founded as performing well in the detection of coronary calcium, coronary stenosis, aortic aneurysm, and giving details on thrombus volume, left atrium segmentation, carotid artery characterization [8]. When considering stroke imaging, 3D CNN is used to flag the areas with a high probability for acute neurologic events in brain CTs with an alarm mechanism for alerting urgent findings and an automated prioritized worklist [7]. Another comparison study has shown the performance of a group of expert radiologists in a level of at or below a 3D CNN cancer risk prediction model [9].
Various diagnostic AI tools have been developed to make histological diagnoses similar to the average pathologists [16]. AI models trained to recognize histopathological images of liver cancer are used to distinguish cancerous tissue from healthy liver tissue [10]. AI has been used to detect polyps from the colonoscopy inputs, detecting GI bleeds or other abnormalities from capsule endoscopy and dysplasia in Barrett’s esophagus and inflammatory bowel disease [11]. Prediction of the severity of BPH and recommending the treatment required for it is another use of AI-based systems [12]. CNNs performing similar to or exceeding dermatologists are used to distinguish dermoscopic images of melanoma from nevi and [15].
3. AI in Surgical Interventions
Robotic-assisted surgeries provide improved ergonomics, 3D visualization, fine instruments, and greater precision for surgeons, and ultimately overcome the limitations of traditional laparoscopies. Combining AI with robotic-assisted surgery is widely applied currently in many surgical fields such as thoracic surgeries, gastroenterological surgeries and gynecological surgeries. For example, it has been more than ten years for robotic myomectomy with reasonable safety and efficacy. A study that evaluated four methods for myomectomy has demonstrated decreased blood loss, smaller scars, shorter length of hospital stay, and fewer postoperative complications in robotic-assisted laparoscopic myomectomy compared to other methods [13].
Research in 2016 has compared intestinal anastomosis metrics such as average suture spacing, anastomosis leaking pressure, number of errors, completion time and the accuracy of reduction in lumen between a supervised autonomous system, manual laparoscopic surgery and robot-assisted surgery. A supervised autonomous system has outperformed the other three methods in some metrics, demonstrating autonomous surgery is feasible [14].
AI Applications During COVID-19 Pandemic
Due to the sudden increase in patients during the COVID-19 pandemic, the workload of healthcare professionals has increased enormously. Providing health services while being themselves safe has become the main challenge of managing the pandemic. But AI can quickly analyze patient-provided symptoms and data generated from medical imaging technologies to diagnose infected cases. Neural networks can extract the visual characteristics of this disease for automated monitoring and treatment of patients without contaminating the healthcare worker. AI can identify vulnerable regions and act accordingly or predict the future course of this disease. By analyzing available data on COVID-19 drug research, AI has already become a powerful tool for diagnostic test design and vaccine development [17].
Ethical Considerations
The possibility of creating thinking machines raises a series of ethical issues. Medical ethics for AI has not explicitly developed in parallel with the vast improvement of AI technologies. But the globally accepted standardized system of ethics regarding the development and use of AI is essential to ensure the protection of private data and eliminate any potential for bias in the decision-making process, therefore establishing trust between systems and users. The protection of privacy is an essential human right that should not be violated in any field. On the other hand, AI has already started an era in which technology replaces the human. The substitution of human beings could damage the human dignity of doctors and nurses. Self-improving artificial intelligence systems could have undesirable consequences when they become aware of human intentions [18].
Future Directions
The miracle of bringing AI to medicine has already come true. Even some achievements of AI in medicine have reduced errors, inefficiencies, and costs, the field still has a relative lack of data and evidence [4]. Therefore, there are many risks of failures and erroneous decisions than those of a single doctor-patient interaction and outcomes. Transferring responsibility of health to machines, except for routine matters with minimal risk, can be overly reliant on science and technology, as human health is more valuable than anything else in human life. Since AI will significantly ingest and process massive data sets quickly, accurately, and inexpensively which may be beyond human capabilities, the proper use of AI will result in high-performance medicine in the future. Yet, the application of artificial intelligence (AI) in medicine has just started.
References
1. He J, Baxter SL, Xu J, Xu J, Zhou X, Zhang K. The practical implementation of artificial intelligence technologies in medicine. Nat Med. 2019 Jan;25(1):30-36. doi: 10.1038/s41591-018-0307-0. Epub 2019 Jan 7. PMID: 30617336; PMCID: PMC6995276. Retrived from https://pubmed.ncbi.nlm.nih.gov/30617336/
2. Kaul V, Enslin S, Gross SA. History of artificial intelligence in medicine. Gastrointest Endosc. 2020 Oct;92(4):807-812. doi: 10.1016/j.gie.2020.06.040. Epub 2020 Jun 18. PMID: 32565184. Retrieved from https://pubmed.ncbi.nlm.nih.gov/325651
3. Mintz Y, Brodie R. Introduction to artificial intelligence in medicine. Minim Invasive Ther Allied Technol. 2019 Apr;28(2):73-81. doi: 10.1080/13645706.2019.1575882. Epub 2019 Feb 27. PMID: 30810430. Retrieved from https://pubmed.ncbi.nlm.nih.gov/30810430/
4. Topol EJ. High-performance medicine: the convergence of human and artificial intelligence. Nat Med. 2019 Jan;25(1):44-56. doi: 10.1038/s41591-018-0300-7. Epub 2019 Jan 7. PMID: 30617339. Retrieved from https://pubmed.ncbi.nlm.nih.gov/30617339/
5. Marchiori C, Dykeman D, Girardi I, Ivankay A, Thandiackal K, Zusag M, Giovannini A, Karpati D, Saenz H. Artificial Intelligence Decision Support for Medical Triage. AMIA Annu Symp Proc. 2021 Jan 25;2020:793-802. PMID: 33936454; PMCID: PMC8075483. Retrieved from https://www.ncbi.nlm.nih.gov/pmc/articles/PMC8075483/
6. Stewart, Jonathon & Sprivulis, Peter & Dwivedi, Girish. Artificial intelligence and machine learning in emergency medicine. Emergency Medicine Australasia. (2018).30. 10.1111/1742-6723.13145. Retrieved from https://pubmed.ncbi.nlm.nih.gov/30014578/
7. Yedavalli VS, Tong E, Martin D, Yeom KW, Forkert ND. Artificial intelligence in stroke imaging: Current and future perspectives. Clin Imaging. 2021 Jan;69:246-254. doi: 10.1016/j.clinimag.2020.09.005. Epub 2020 Sep 21. PMID: 32980785. Retrieved from https://pubmed.ncbi.nlm.nih.gov/32980785/
Related Article: Digital Transformation in the Healthcare Industry
8. Itchhaporia D. Artificial intelligence in cardiology. Trends Cardiovasc Med. 2020 Nov 23:S1050-1738(20)30151-1. doi: 10.1016/j.tcm.2020.11.007. Epub ahead of print. PMID: 33242635. Retrieved from https://pubmed.ncbi.nlm.nih.gov/33242635/
9. Khemasuwan D, Sorensen JS, Colt HG. Artificial intelligence in pulmonary medicine: computer vision, predictive model and COVID-19. Eur Respir Rev. 2020 Oct 1;29(157):200181. doi: 10.1183/16000617.0181-2020. PMID: 33004526; PMCID: PMC7537944. Retrieved from https://www.ncbi.nlm.nih.gov/pmc/articles/PMC7537944/
10. Ivanics T, Patel MS, Erdman L, Sapisochin G. Artificial intelligence in transplantation (machine-learning classifiers and transplant oncology). Curr Opin Organ Transplant. 2020 Aug;25(4):426-434. doi: 10.1097/MOT.0000000000000773. PMID: 32487887. Retrieved from https://pubmed.ncbi.nlm.nih.gov/32487887/
11. Kochhar GS, Carleton NM, Thakkar S. Assessing perspectives on artificial intelligence applications to gastroenterology. Gastrointest Endosc. 2021 Apr;93(4):971-975.e2. doi: 10.1016/j.gie.2020.10.029. Epub 2020 Nov 2. PMID: 33144237. Retrieved from https://pubmed.ncbi.nlm.nih.gov/33144237/
12. Shah M, Naik N, Somani BK, Hameed BMZ. Artificial intelligence (AI) in urology-Current use and future directions: An iTRUE study. Turk J Urol. 2020 Nov;46(Supp. 1):S27-S39. doi: 10.5152/tud.2020.20117. Epub 2020 May 27. PMID: 32479253; PMCID: PMC7731952. Retrieved from https://pubmed.ncbi.nlm.nih.gov/32479253/
Related Article: Cybersecurity in Medicine – An overview
13. Wang R, Pan W, Jin L, Li Y, Geng Y, Gao C, Chen G, Wang H, Ma D, Liao S. Artificial intelligence in reproductive medicine. Reproduction. 2019 Oct;158(4):R139-R154. doi: 10.1530/REP-18-0523. PMID: 30970326; PMCID: PMC6733338. Retrieved from https://www.ncbi.nlm.nih.gov/pmc/articles/PMC6733338/
14. Etienne H, Hamdi S, Le Roux M, Camuset J, Khalife-Hocquemiller T, Giol M, Debrosse D, Assouad J. Artificial intelligence in thoracic surgery: past, present, perspective and limits. Eur Respir Rev. 2020 Aug 18;29(157):200010. doi: 10.1183/16000617.0010-2020. PMID: 32817112. Retrieved from https://pubmed.ncbi.nlm.nih.gov/32817112/
15.Young AT, Xiong M, Pfau J, Keiser MJ, Wei ML. Artificial Intelligence in Dermatology: A Primer. J Invest Dermatol. 2020 Aug;140(8):1504-1512. doi: 10.1016/j.jid.2020.02.026. Epub 2020 Mar 27. PMID: 32229141. Retrieved from https://pubmed.ncbi.nlm.nih.gov/32229141/
16. Rakha EA, Toss M, Shiino S, Gamble P, Jaroensri R, Mermel CH, Chen PC. Current and future applications of artificial intelligence in pathology: a clinical perspective. J Clin Pathol. 2020 Aug 6:jclinpath-2020-206908. doi: 10.1136/jclinpath-2020-206908. Epub ahead of print. PMID: 32763920. Retrieved from https://pubmed.ncbi.nlm.nih.gov/32763920/
17. Vaishya R, Javaid M, Khan IH, Haleem A. Artificial Intelligence (AI) applications for COVID-19 pandemic. Diabetes Metab Syndr. 2020 Jul-Aug;14(4):337-339. doi: 10.1016/j.dsx.2020.04.012. Epub 2020 Apr 14. PMID: 32305024; PMCID: PMC7195043. Retrieved from https://www.ncbi.nlm.nih.gov/pmc/articles/PMC7195043/
18. Keskinbora KH. Medical ethics considerations on artificial intelligence. J Clin Neurosci. 2019 Jun;64:277-282. doi: 10.1016/j.jocn.2019.03.001. Epub 2019 Mar 14. PMID: 30878282. Retrieved from https://pubmed.ncbi.nlm.nih.gov/30878282/