Data Science in Healthcare: Why Is It a Big Deal?
Healthful Vitality | 02/01/2023 | Data Science in Healthcare: Why Is It a Big Deal?
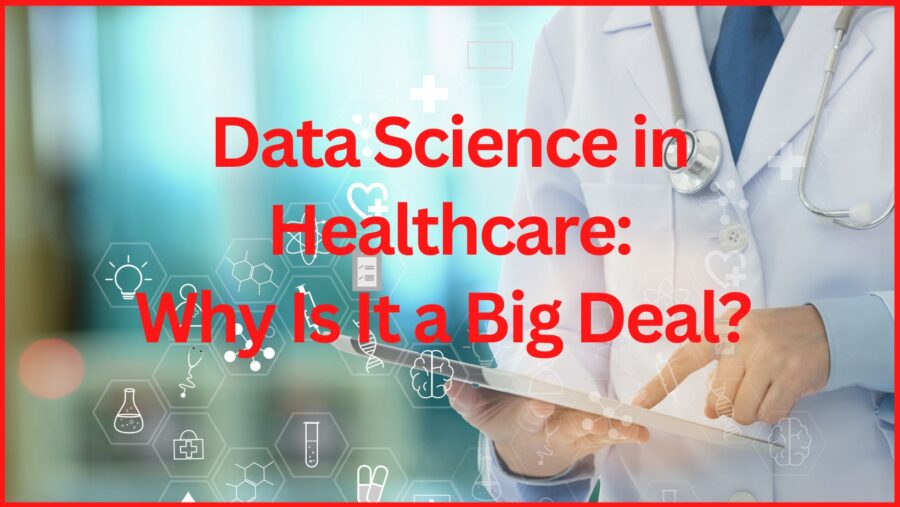
Data science in healthcare refers to the use of data analysis and machine learning techniques to improve health outcomes, increase efficiency in healthcare delivery, and support healthcare professionals’ decision-making. This involves collecting and analyzing large amounts of healthcare data, including electronic medical records, claims data, and genomics data, to gain insights and make predictions that can inform clinical practice, public health policy, and the development of new treatments and technologies. Data science applications in healthcare include personalized medicine, population health management, disease diagnosis and prognosis, and drug development.
Notably, data science has become a required field in recent years. The exponential growth of data and the increasing availability of computing power and advanced algorithms have enabled data scientists to develop new insights and solve complex problems in various industries, including healthcare, finance, retail, and more. As a result, data science is transforming how organizations make decisions, develop new products and services, and improve customer experiences. As the world continues to generate more data, the importance of data science is only expected to grow.
Therefore, data science has the potential to transform healthcare organizations significantly. By leveraging large amounts of data and advanced analytics techniques, data science can help healthcare organizations make more informed decisions, improve patient outcomes, and increase efficiency.
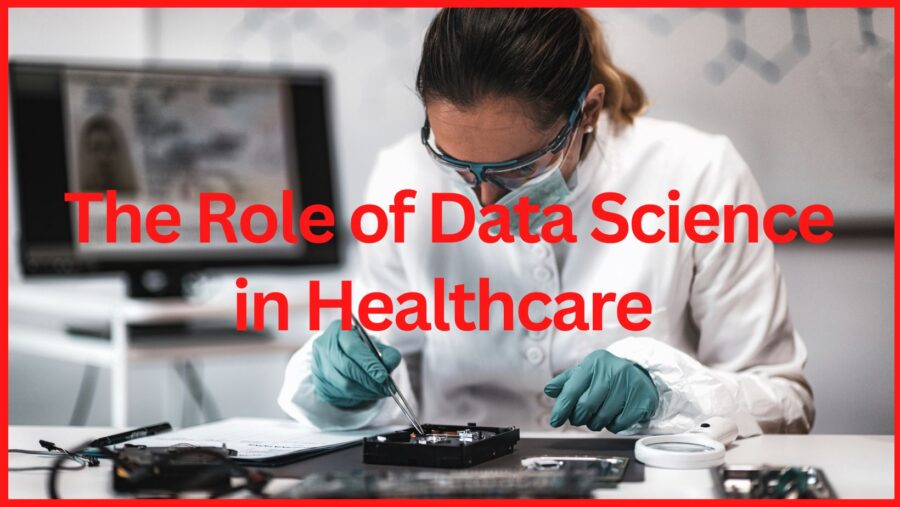
The role of data science in healthcare
Some specific roles of data science in healthcare include:
- Predictive Modeling. Data science can help create predictive models that identify patients at risk for certain conditions, predict disease progression, and inform treatment decisions.
- Clinical Decision Support. Data science can provide healthcare professionals with real-time decision support, using algorithms to analyze patient data and provide personalized recommendations.
- Electronic Health Record Analysis. Data science can assist in analyzing electronic health records to identify trends and improve patient outcomes.
- Personalized Medicine. Data science can support the development of customized treatment plans based on a patient’s characteristics, including genetics and lifestyle factors.
- Population Health Management. Data science can assist in identifying and targeting health issues in populations, leading to improved public health outcomes.
- Drug Discovery and Development. Data science allows to analyze large amounts of data on drug efficacy and safety, leading to faster and more effective drug development.
- Fraud Detection. Data science can assist in identifying and preventing healthcare fraud, improving the efficiency and cost-effectiveness of the healthcare system.
- Patient Engagement. Data science can assist in developing tools and platforms that engage patients and improve their understanding of their health and treatment options.
Benefits of data science in healthcare
The benefits of data science in healthcare are numerous and can significantly impact patient outcomes and the overall healthcare system. Some of the key benefits include:
- Improved Patient Outcomes. By analyzing large amounts of patient data, data science can help identify patterns and relationships that can inform treatment decisions and improve patient outcomes.
- Efficient and Effective Decision-making. Data science can provide healthcare professionals with insights that can support informed decision-making and lead to more efficient and effective use of resources.
- Personalized Medicine. Data science uses to develop personalized treatment plans based on a patient’s unique characteristics, genetics, and lifestyle.
- Improved Population Health. Data science can identify and target population health issues, leading to improved public health outcomes.
- Cost Savings. By improving the healthcare system’s efficiency and reducing unnecessary tests and treatments, data science can lead to significant cost savings.
- Improved Drug Development. Data science helps to analyze large amounts of data on drug efficacy and safety, conducting more rapid and beneficial drug development.
- Enhanced Patient Engagement. Data science uses to develop tools and platforms that engage patients and improve their understanding of their health and treatment options.
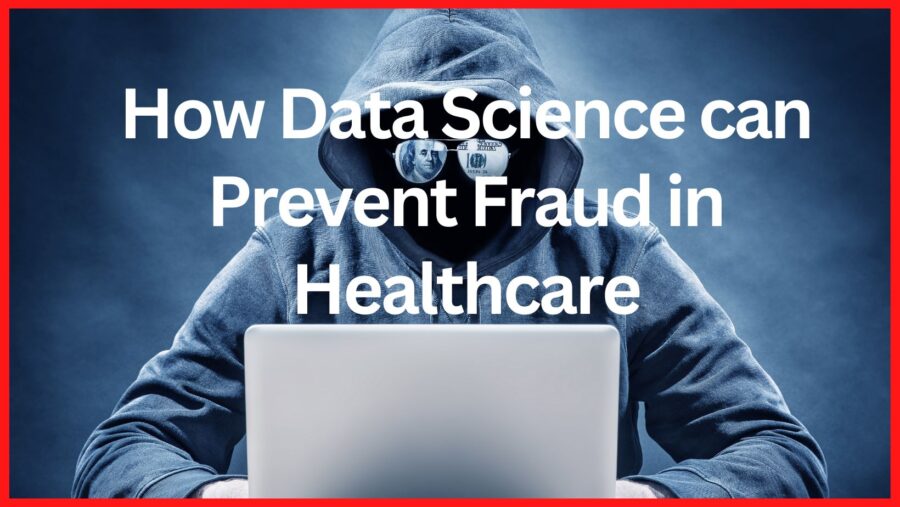
How data science can prevent fraud in healthcare
Data science can play a crucial role in preventing fraud in the healthcare industry by using advanced analytical techniques to identify fraudulent activities. Here are some ways data science can help prevent healthcare fraud:
- Data Mining and Analytics. Data scientists can analyze large amounts of healthcare data to identify patterns and anomalies that may indicate fraud. This includes analyzing claims data, provider information, and patient demographic information.
- Predictive Modeling. Machine learning algorithms can be trained on historical data to identify the characteristics of fraudulent claims. These models can then detect fraud in real time by scoring new claims and flagging those likely to be fraudulent.
- Network Analysis. By analyzing relationships between healthcare providers, patients, and insurance companies, data scientists can identify clusters of suspicious activity and potential fraud rings.
- Rule-based Systems. Fraud detection systems designed help to flag claims that violate specific rules, such as excessive billing for a particular service or submitting claims for patients who do not live in the area.
- Natural Language Processing (NLP). NLP techniques uses to process and analyze large amounts of unstructured data, such as physician notes and patient medical records, to identify signs of potential fraud.
History of healthcare fraud
Healthcare fraud has been a persistent issue throughout history. Notably, with fraudulent activities recorded as far back as the early 19th century. In the early days of healthcare fraud, scams were often simple and involved false billing for services or supplies that were not provided. However, as healthcare systems have become more complex, so have the methods of fraud.
In the 20th century, technological advances and the increasing use of insurance and government-funded healthcare programs created new opportunities for healthcare fraud. In addition, the growth of managed care in the 1980s and 1990s made it easier for fraudsters to exploit the system. Particularly, the increased volume of claims made it more difficult to detect fraud.
The introduction of the Internet and the increasing use of electronic health records in the 21st century have created new opportunities for fraud. Additionally, they also created new challenges for detecting and preventing it. The U.S. government has recently taken steps to combat healthcare fraud. These steps include creating the Healthcare Fraud Prevention and Enforcement Action Team (HEAT) in 2009. Despite these efforts, healthcare fraud continues to be a significant problem. The U.S. Department of Justice estimates that healthcare fraud costs the government and private payers billions yearly.
Data science can significantly enhance the ability of healthcare organizations to detect and prevent fraud. By utilizing data-driven techniques and advanced analytics, organizations can improve the accuracy of their fraud detection efforts, reduce their financial losses, and protect their patients from potential harm.
Data science in healthcare and predictive analytics
Predictive analytics is a crucial area of focus for data science in healthcare. It is because predictive analytics has the potential to improve patient outcomes and reduce healthcare costs. Predictive analytics uses data, statistical algorithms, and machine learning techniques to identify the likelihood of future results based on historical data. In healthcare, predictive analytics can be used for a variety of purposes, including:
- Risk Assessment: Predictive analytics can assess a patient’s risk of developing a disease or condition. This information can then be used to inform clinical decision-making and provide targeted preventive care.
- Patient Readmissions: Predictive analytics can identify patients at high risk of readmission after being discharged from the hospital. This information is useful to develop interventions to reduce readmissions and improve patient outcomes.
- Disease Management: Predictive analytics can identify patients at high risk of developing chronic conditions, such as diabetes or heart disease. This information provides targeted care and disease management programs to prevent or delay the condition’s onset.
- Predictive Maintenance: Predictive analytics can be used to identify medical equipment that is likely to fail, allowing for proactive maintenance and reducing the likelihood of equipment failure during critical procedures.
- Fraud Detection: Predictive analytics assists to determine fraudulent activities in the healthcare system, such as false billing for services that were not delivered.
Predictive analytics is a powerful tool for data science in healthcare and can potentially transform care delivery. By leveraging the power of data and advanced analytics, healthcare organizations can make informed decisions, improve patient outcomes, and reduce healthcare costs.
What size of hospitals can use data science in healthcare?
Hospitals of all sizes can use data science, regardless of the number of patients they serve or the complexity of their operations. The benefits of using data science in healthcare are not limited to large hospitals with extensive resources. Still, they can also be realized by smaller hospitals and clinics that serve a local community.
Smaller hospitals can use data science to improve patient outcomes by leveraging the power of predictive analytics, For example, to identify patients who are at high risk of readmission or to develop targeted disease management programs.
Similarly, larger hospitals can benefit from data science by using it to improve the efficiency and accuracy of their operations. For example, by automating the data entry process or by integrating data from multiple sources to gain a complete picture of a patient’s health.
In short, data science has the potential to transform the healthcare industry and improve patient outcomes, regardless of the size of the hospital. By leveraging the power of data and advanced analytics, healthcare organizations of all sizes can make informed decisions, improve the overall delivery of care, and reduce healthcare costs.
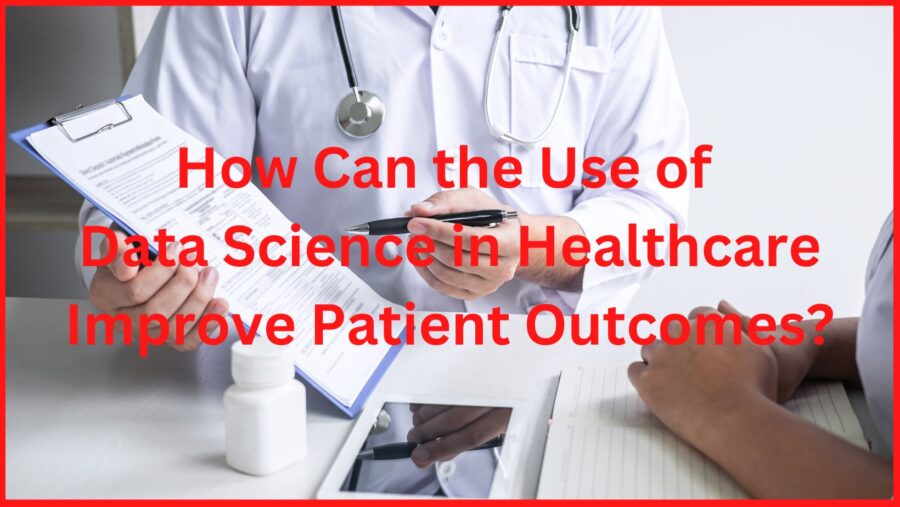
How can the use of data science in healthcare improve patient outcomes?
Data science in healthcare has the potential to significantly improve patient outcomes. Here are some ways data science can help improve patient outcomes:
- Predictive Analytics: Data science assists to analyze patient data and identify those who are at high risk of developing certain conditions or experiencing adverse outcomes. This information allows to develop targeted interventions and preventative measures that can improve patient outcomes.
- Clinical Decision Support: Data science helps to develop decision support tools that provide real-time information about patient conditions and treatments. This information helps healthcare providers make informed decisions about patient care and improve patient outcomes.
- Patient Monitoring: The use of data science allows to develop patient monitoring systems that track patients’ health status and alert healthcare providers to changes in their condition. This information is useful to intervene early and prevent adverse outcomes.
- Personalized Medicine: Data science helps to analyze patient data and develop personalized treatment plans based on a patient’s unique characteristics and health history. This approach can improve patient outcomes by ensuring that patients receive the most effective treatments for their specific needs.
- Improved Care Coordination: The use of data science helps to integrate data from multiple sources and develop a more complete picture of a patient’s health. This information helps to coordinate care between healthcare providers and ensure that patients receive the most appropriate and effective treatments.
- Help with Patient Readmissions: Data science helps to identify patients who are at high risk of readmission after being discharged from the hospital. This information allows to develop targeted interventions to reduce readmissions and improve patient outcomes.
By leveraging the power of data and advanced analytics, healthcare organizations can make informed decisions, improve the overall delivery of care, and ultimately improve patient outcomes.
How doctors can be trained to use data science in healthcare?
Doctors can be trained to use data science in healthcare through a combination of education, training programs, and hands-on experience. Here are some steps that can be taken to train doctors to use data science in healthcare:
- Formal Education: Doctors can be trained in data science through formal educational programs, such as graduate-level courses or certificate programs. These programs can provide doctors with a strong foundation in the concepts and techniques of data science, including data analysis, machine learning, and predictive analytics.
- Workshops and Training Programs: Workshops and training programs can provide doctors with hands-on experience using data science tools and techniques in a healthcare setting. These programs can be designed to meet the specific needs of doctors, such as training in the use of data science for predictive analytics or for patient monitoring.
- Collaboration with Data Scientists: Doctors can also benefit from working closely with data scientists, who can provide support and guidance in using data science tools and techniques. This collaboration can help doctors develop their data science skills and apply them to real-world healthcare problems.
- On-the-Job Training: Doctors can also gain experience using data science in healthcare by working on projects that involve data analysis and machine learning. These projects can allow doctors to apply their skills in a real-world setting and gain hands-on experience using data science tools and techniques.
Training doctors to use data science in healthcare can help to improve patient outcomes and to advance the overall delivery of care. By investing in the education and training of doctors, healthcare organizations can ensure that they are well-equipped to leverage the power of data and advanced analytics to improve patient outcomes.
Is it beneficial for hospital nurses to learn data science?
It can be highly beneficial for hospitals and nurses to learn data science. Here are some ways data science can help improve the practice of nursing:
- Improved Patient Outcomes: By analyzing patient data and identifying those at high risk of developing certain conditions, data science can help nurses make informed decisions about patient care and improve patient outcomes.
- Evidence-Based Practice: Data science can help nurses make decisions based on the latest research and evidence-based practice. Nurses can use this data to inform treatment plans and improve patient outcomes.
- Improved Efficiency: Data science allows to automate specific tasks and processes in healthcare, such as data entry and tracking patient health status. This can help nurses to work more efficiently and effectively, allowing them to spend more time with patients.
- Better Patient Monitoring: Data science helps to develop patient monitoring systems that track patients’ health situations and can provide nurses with an alert about changes in patient’s condition. This information helps nurses to intervene early and prevent adverse outcomes.
- Improved Care Coordination: Data science can integrate data from numerous sources and conceive a complete picture of a patient’s health. Nurses can use this information to coordinate care between healthcare providers and ensure patients receive exceptional care.
By learning data science, hospitals and nurses can improve care delivery, make informed decisions, and ultimately improve patient outcomes.
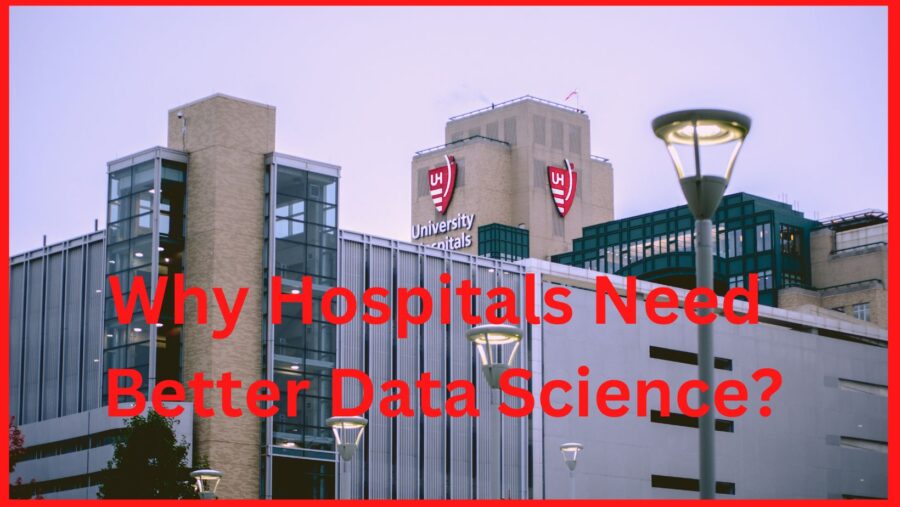
Why hospitals need better data science?
There are several reasons why hospitals need better data science:
- Improved Patient Outcomes: Data science uses to examine patient data and determine those at high risk of developing certain conditions or experiencing adverse outcomes. Healthcare providers can use this information to develop targeted interventions and preventative measures to improve patient outcomes.
- Evidence-Based Practice: Data science can help healthcare providers make informed decisions established on the latest research and evidence-based practice. Healthcare providers can use this information to inform treatment plans and enhance patient outcomes.
- Better Resource Allocation: Data science helps to analyze resource utilization data and helps hospitals allocate resources more effectively. A better resource allocation can enhance hospital operations’ efficiency and reduce waste.
- Improved Quality of Care: Data science allows to analyze data on patient outcomes and identify areas for improvement in the quality of care. Healthcare providers can use this information to implement quality improvement initiatives and improve patient outcomes.
- Better Clinical Decision Making: Data science helps to design decision-support tools that provide real-time information about patient conditions and treatments. This information allows healthcare providers in making informed decisions about patient care and improve patient outcomes.
Integrating data science into hospital operations allows healthcare providers to make informed decisions, improve patient outcomes, and deliver high-quality care more efficiently.
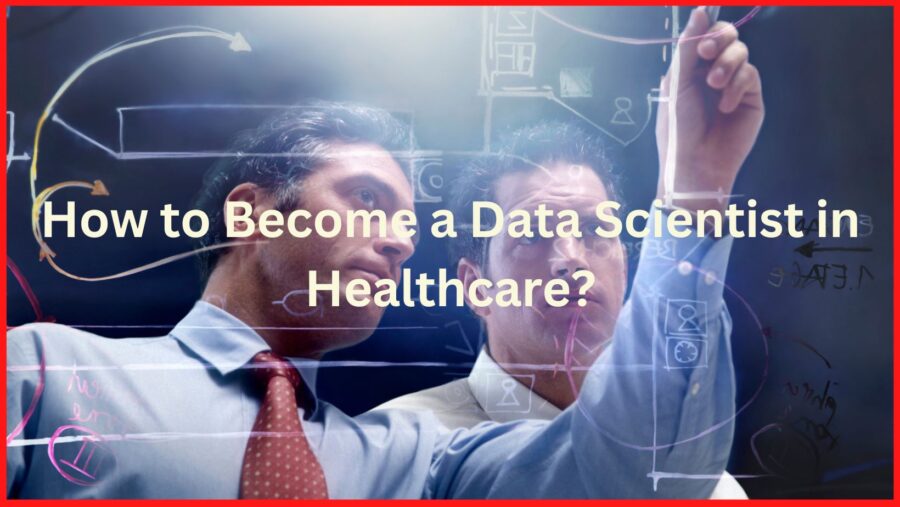
How to become a Data Scientist in healthcare?
To become a data scientist in healthcare, one can follow these steps:
- Earn a Degree in a Relevant Field: A strong foundation in mathematics, statistics, and computer science is essential for a career in data science. One can earn a bachelor’s or master’s degree in these fields or a related field such as biostatistics, bioinformatics, or health informatics.
- Gain Experience: Practical experience is essential for success as a data scientist. One can gain experience through internships, volunteering, or working in entry-level roles in the healthcare or technology industries.
- Develop Technical Skills: Data scientists need a robust set of technical skills, including proficiency in programming languages such as Python, R, and SQL, as well as experience with data visualization and machine learning algorithms.
- Familiarize Yourself with Healthcare Data: Data scientists in healthcare need to have a strong understanding of healthcare data, including electronic health records (EHRs), claims data, and clinical data. They also need to understand the regulatory and privacy requirements related to healthcare data.
- Stay Current with Industry Trends: The field of data science is constantly evolving, and data scientists in healthcare need to stay up-to-date with the latest technologies and best practices. This can be done through ongoing education and professional development opportunities.
- Build a Network: Networking with other professionals in the field can help one learn about job opportunities, industry trends, and best practices. One can join professional organizations, attend conferences and events, and connect with others on professional networking sites such as LinkedIn.
By following these steps and continuously developing your skills and knowledge, you can become a successful data scientist in healthcare and positively impact patient outcomes and the healthcare industry as a whole.
Data science in healthcare vs. data analysis in healthcare
In general, data science in healthcare and data analysis in healthcare are similar but distinct fields with different goals and approaches.
Data analysis in healthcare involves collecting, organizing, and interpreting healthcare data to inform decisions and improve patient outcomes. This may involve simple statistical analysis, such as calculating averages or determining correlation. This may also involve more complex data analysis, such as predictive modeling or network analysis.
On the other hand, data science in healthcare encompasses a broader range of activities and approaches, including data analysis. Healthcare data scientists use computer science, statistics, and domain expertise to extract insights from healthcare data, develop predictive models, and inform clinical decision-making.
In summary, while data analysis in healthcare is a subset of data science in healthcare, data science encompasses a more comprehensive approach to working with healthcare data, involving the use of advanced data analysis techniques and machine learning algorithms, as well as a deep understanding of the healthcare domain.
Data science in healthcare vs. big data in healthcare
Generally speaking, data science in healthcare and big data in healthcare are related but distinct fields.
Data science in healthcare involves using statistical and computational methods to extract insights and make predictions from healthcare data. This includes using data analysis techniques, machine learning algorithms. And also includes other methods to develop predictive models and inform clinical decision-making.
Big data in healthcare refers to the large and complex sets of healthcare data that are generated from various sources. For example, sources like electronic medical records (EMRs), medical imaging, and wearable devices. Big data has the potential to provide a more complete picture of patient health. However, it requires advanced computational tools and techniques to manage and analyze effectively.
In summary, data science in healthcare uses big data as a source of information. In contrast, big data in healthcare refers to the vast amounts of healthcare data that are generated and stored. Data science in healthcare leverages big data to develop predictive models. These models enhance clinical decision-making and improve patient outcomes.
How does big data help healthcare?
Big data can help healthcare in several ways:
- Improved Patient Outcomes. Big data helps to analyze patient records, medical histories, and outcomes data to identify patterns and predict future outcomes. This information allows to develop more effective treatment plans, improve patient outcomes, and reduce readmissions.
- Enhanced Disease Surveillance. Big data helps to monitor disease outbreaks, track the spread of infections, and identify high-risk populations. This information allows to develop targeted prevention and control measures to reduce the impact of disease outbreaks.
- Improved Drug Development. Big data allows healthcare providers to analyze large amounts of information. For example, information about drug efficacy and side effects, which can inform the development of new, more effective drugs.
- Personalized Medicine. Big data helps to develop tailored treatment plans based on an individual’s medical history, genetic makeup, and lifestyle factors.
- Streamlined Operations. Big data allows to optimize hospital operations. For example, scheduling, resource allocation, and supply chain management, reducing costs and improving efficiency.
- Improved Public Health. Big data is useful to monitor and improve public health outcomes. For example, reducing health disparities and improving access to care.
Overall, big data has the potential to revolutionize the healthcare industry. Notably, by providing more accurate and actionable information to support better decision making, improve patient outcomes, and reduce costs.
What are the top universities offering data science in healthcare?
Here are some top universities that offer programs or courses in data science and healthcare:
- Harvard University, Cambridge, MA
- Stanford University, Stanford, CA
- Johns Hopkins University, Baltimore, MD
- University of California, Berkeley, CA
- Columbia University, New York, NY
- Massachusetts Institute of Technology (MIT), Cambridge, MA
- University of Michigan, Ann Arbor, MI
- University of Pennsylvania, Philadelphia, PA
- Duke University, Durham, NC
- Northwestern University, Evanston, IL
These universities offer various degree programs in data science, health informatics, biostatistics, and related fields. Some of them also offer specialized courses and certificates in data science for healthcare. Research the specific programs and courses offered by these universities and choose the one that best meets your career goals and interests.
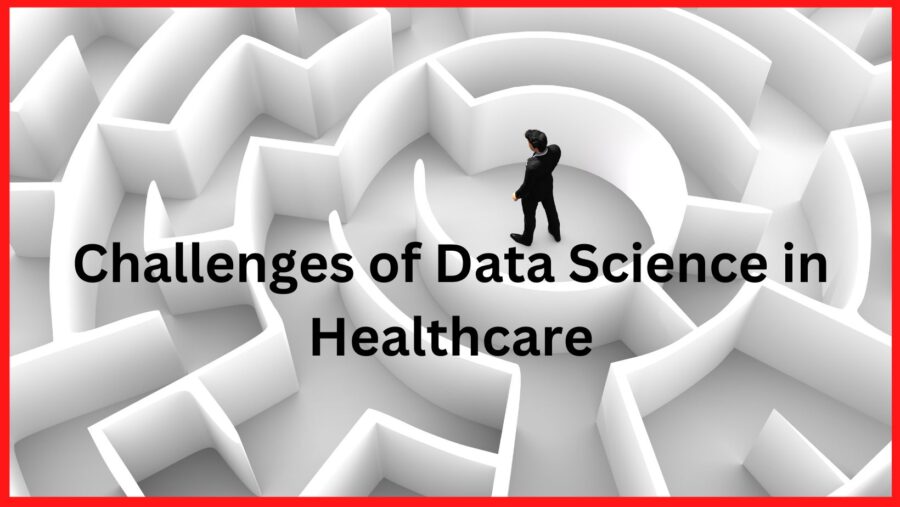
Challenges of data science in healthcare
There are several challenges that need to be overcome to realize the full potential of data science in healthcare:
- Data Quality and Availability. Healthcare data is often incomplete, inconsistent, and unreliable, making it challenging to extract meaningful insights.
- Data Privacy and Security. Healthcare data is sensitive and confidential and must be protected against unauthorized access and breaches.
- Integration with Clinical Workflows. Data science solutions must be integrated into clinical workflows in a user-friendly way that does not disrupt existing processes.
- Data Sharing and Collaboration. Healthcare data is generated and stored by multiple stakeholders, including patients, hospitals, and insurance companies. Ensuring that data can be shared and used effectively across these different groups is critical to the success of data science initiatives.
- Regulation and Standards. Healthcare is heavily regulated, and data science solutions must adhere to strict privacy and security regulations to be adopted and used effectively.
- Technical Skills. Healthcare organizations often need more technical skills and expertise to implement data science solutions effectively.
- Funding and Resources. Implementing data science solutions in healthcare can be expensive and require significant infrastructure, hardware, and personnel investment.
Despite these challenges, the potential benefits of data science in healthcare make it an area of growing interest and investment. Addressing these challenges will be essential to realizing the full potential of data science in healthcare and improving patient outcomes.
Conclusion
Data science in healthcare involves the application of data analysis and machine learning techniques to improve patient outcomes. It makes the healthcare system more efficient. It involves leveraging large amounts of data generated from different sources. These sources include electronic health records, medical devices, and clinical trials. The use of data science in healthcare aims to identify patterns, make predictions, and support decision-making. Thus, data science has great potential to transform healthcare industry. Notably, by enabling personalized medicine, improving patient outcomes, reducing costs, and helping early disease detection and prevention. Despite challenges such as data privacy, integration with clinical workflows, and regulatory compliance, data science is becoming increasingly important in healthcare. And data science expected to continue to play a significant role in transforming the industry.
Related Articles:
10 Strong Benefits of Digital Transformation in Hospitals
The Application of Artificial Intelligence (AI) in Medicine
Digital Transformation in the Healthcare Industry
Immunotherapy Weds Artificial Intelligence: All Cancer Patients are Invited
The Top 10 Best Medical Software of 2022
Health e-Messenger – An Evolutionary Way to Provide Healthcare
References:
https://www.sas.com/en_us/insights/analytics/predictive-analytics.html
https://blog.lyrise.ai/lyguide-series-what-is-ai-rd
https://365datascience.com/career-advice/how-to-become-a-data-scientist-in-healthcare/